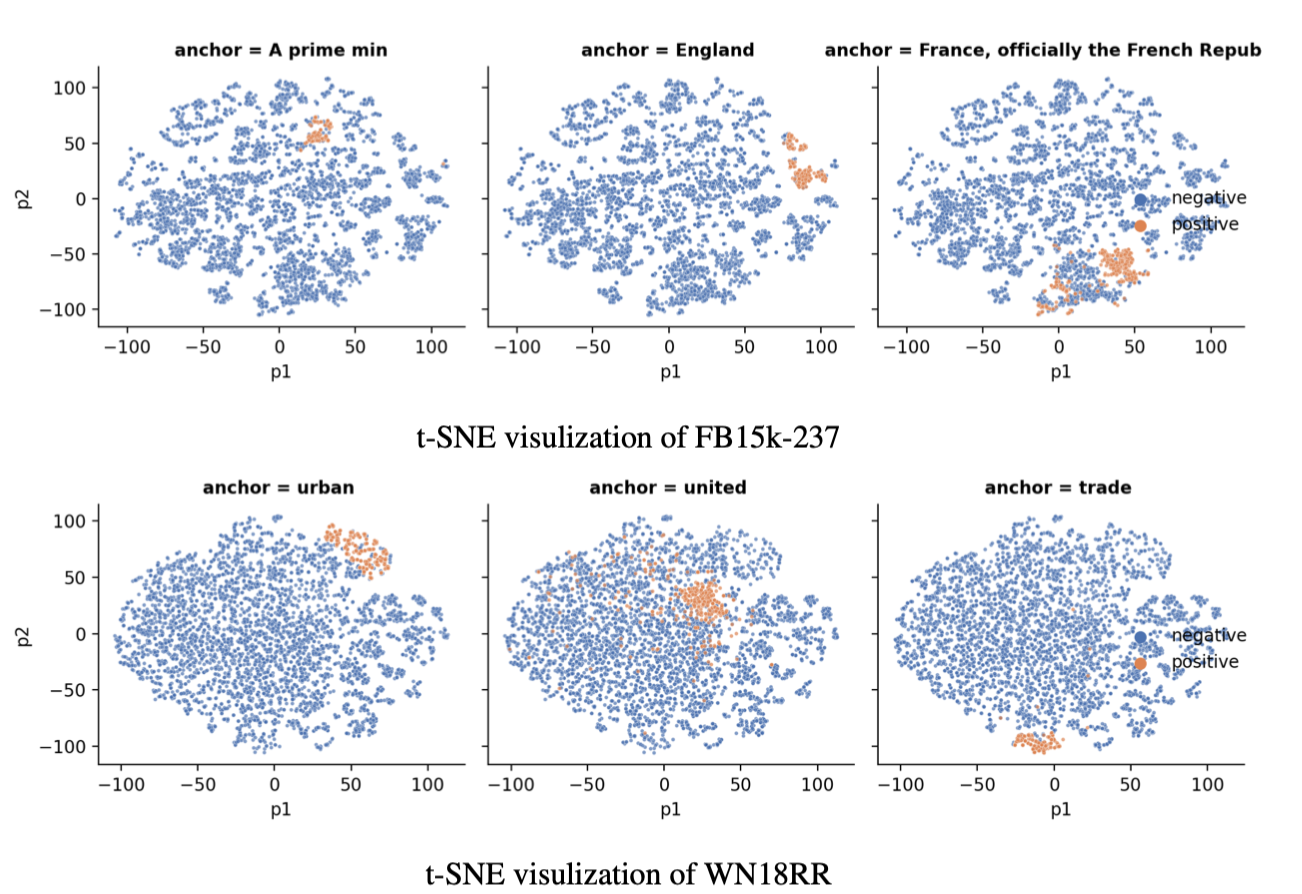
Knowledge graph embedding [Arxiv preprint]
I am currently working on developing a machine-learning model that utilizes non-labeled data. My research is focused on knowledge graphs, which are comprised of triples containing factual information about the world (head entity, relationship, tail entity). However, due to the incompleteness of knowledge graphs, I am pursuing the task of knowledge graph completion. To address this problem, I approach it as a fill-in-the-blank challenge, where missing parts of the knowledge graph are predicted using the available data. To improve the accuracy of these predictions, have proposed a debiased contrastive knowledge graph embedding technique. This involves generating non-factual triples to contrast with the factual ones, resulting in a more accurate model. I am proud to say that our method has achieved state-of-the-art results in knowledge graph completion.